The impact of Industry 4.0 on automotive manufacturing
Last updated on February 5th, 2024
Much of what was learned from Henry Ford’s first moving assembly line in 1913, and the resulting mass-production movement, paved the way for smart manufacturing in the automotive industry leveraging Industry 4.0 technologies.
That first assembly line enabled an entire automobile to be produced in 1 hour and 33 minutes, rather than the typical 12+ hours, by optimizing processes while retaining or even improving product quality. Ford also built machines to stamp out parts automatically – and faster than any human could. The resulting efficiency gains from his assembly line and automated machines helped lower the cost of cars and make them more accessible to the masses.
Ford’s methods developed during the age of mass production, or 2nd Industrial Revolution, set the ball rolling for further automation and the dawning and maturity of computerization in the 3rd Industrial Revolution.
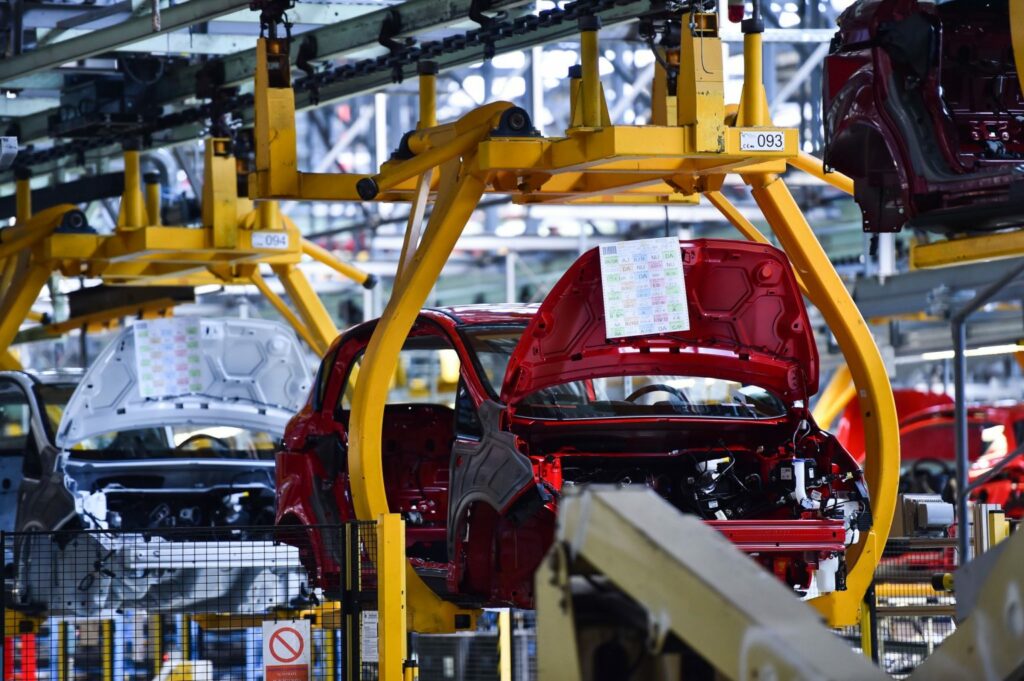
Today, we are in the midst of the 4th Industrial Revolution, or Industry 4.0, which is powered by cyber-physical systems, such as the Internet of Things (IOT), artificial intelligence (AI), machine learning (ML), virtual reality (VR) and augmented reality (AR).
Industry 4.0 continues to contribute to the evolution of automotive manufacturing technologies, for the sake of optimizing processes, realizing economies of scale, complementing or reducing the work done by people, increasing efficiencies, and improving product quality.
Let’s take a look at how some of these Industry 4.0 technologies are impacting the automotive industry. But first we need to recognize how important data is to the success of any of these technologies.
The importance of data in modern precision manufacturing
The automotive industry generates enormous amounts of data. Thanks to Industry 4.0 innovations, such as machine learning (ML) and artificial intelligence (AI), masses of current and historical data can be quickly and accurately analyzed. The end goal is to provide manufacturers with insights to develop better processes, deal with production issues in real time, predict problems before they occur, improve product quality and reduce waste.
Because these technologies are connected, they can share insights from the data collected and be used to make improvements to the supply chain, including distribution optimization and prototype production. To learn more about building a data science strategy, read our blog here.
Key Industry 4.0 technologies that impact the automotive industry
For leading automakers, the main objective for implementing Industry 4.0 technologies is to design a more efficient, precise, and cost-effective manufacturing process that builds quality products. Here are the key technologies they draw upon.
The Industrial Internet of Things
The Industrial Internet of Things (IIoT) on the production line refers to interconnected sensors and other devices that can communicate with each other and exchange data in real time.
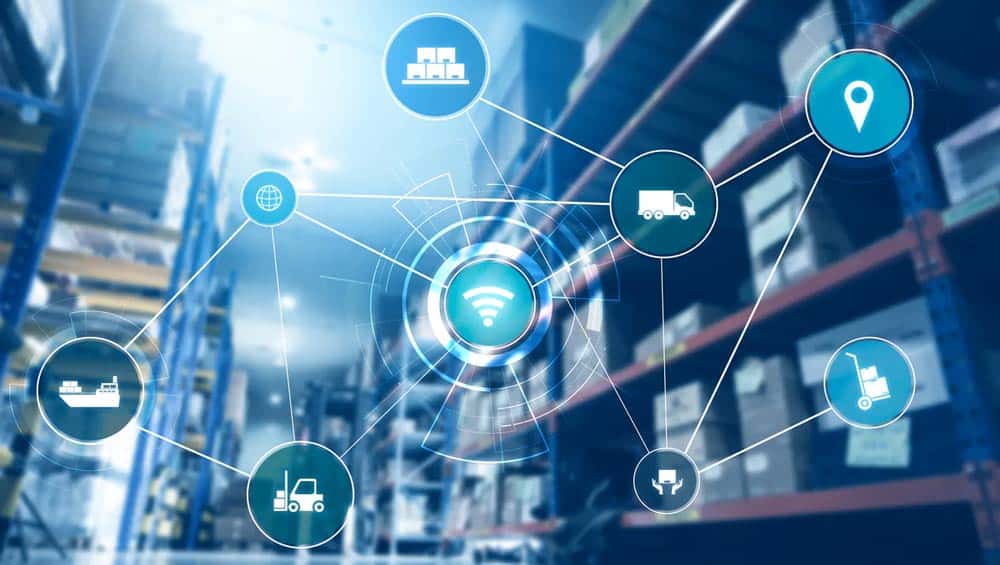
IIoT smart sensors are instrumental to Industry 4.0 smart factories. They enable manufacturers to:
- Lower production costs and run environmentally sustainable operations, using sensors on machines that can measure and reduce excess energy consumption
- Improve production output by optimizing asset-tracking methods and automating inventory management systems that generate alerts when stock levels are low
- Decrease downtime, using smart sensors and cloud-based manufacturing optimization software to identify potential equipment failures before they happen
- Reduce scrapping and reworking of parts, by leveraging real-time predictive quality control analytics to identify problems that could lead to faults
The automotive industry is increasingly turning to the IIoT–enabled smart factory model to help optimize production efficiencies and product throughput. Research has shown that manufacturers can realize annual productivity gains of between 2.8% and 4.4% by implementing smart factory initiatives.
Artificial intelligence and machine learning technologies
Machine learning models, powered by artificial intelligence, can predict anomalies and uncover previously hidden correlations and patterns in manufacturing data, delivering a deeper understanding of the production process and product quality insights. It is estimated that the value of the automotive AI market will reach $19.1 billion by 2030, up from $5.58 billion in 2022, representing a CAGR of 23.3%.
The versatility of ML/AI solutions can also benefit manufacturers beyond the factory floor, by empowering them with the means to collect and analyze data from all relevant departments and 3rd-party partners and use these insights to design and deploy solutions that benefit their bottom line.
In the automotive industry, the mountains of data available needs to be collected, sorted, and analyzed before any value can be derived from it. An ML-powered manufacturing data analytics solution can quickly and accurately analyze all of the information before, during, and after the manufacturing process to deliver actionable insights. Manufacturers rely on such solutions to:
- Automate root cause analysis to locate and understand the core reasons for defects with greater speed and accuracy, resolve problems proactively, and eliminate disruptive impacts on production
- Detect abnormalities and observe how the data has changed over time which can predict product defects and improve throughput and quality while reducing scrap and rework
- Connect real-time production and quality data from across the shop floor and even from different facilities to analyze
ML/AI solutions not only perform the tedious tasks of monitoring, data processing, and analytics, they also save costs through data-driven optimizations.
Example: A major Tier-1 supplier in the automotive industry partnered with Acerta to reduce product fallout and rework in their axle assembly production. With over 200 signals generated by each assembled unit and multiple potential failure modes, the challenge was to pinpoint the sources of failures effectively. Acerta’s LinePulse platform employed machine learning models to identify key signals predicting backlash failures, streamlining the identification of failure causes and relationships within the production process. This approach replaced traditional tolerance stack calculations with more accurate ML models. The solution achieved a 65% reduction in failure and rework rates, significantly improving first-time yield and reducing costs for the client, leading to its adoption across global facilities.
Virtual reality and augmented reality
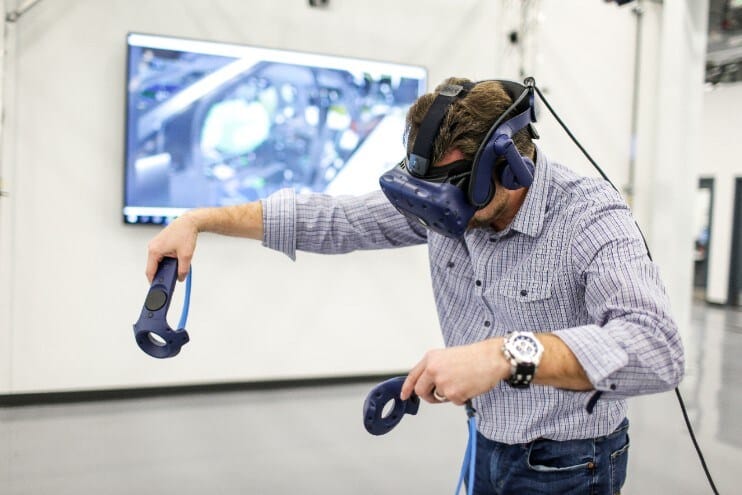
Virtual reality is a completely virtual environment, and users are controlled by the operating system. VR delivers an exact simulation of a product, process, or production plant and presents an immersive display of their operations.
Augmented reality, on the other hand, is used by manufacturers in a real-world setting. For instance, engineers use augmented reality to manipulate their presence in the real world to visualize the various steps of a procedure, including maintenance tasks, assembly processes, and quality controls.
Augmented reality enhances both the virtual world and the real world, whereas virtual reality only enhances a fictional reality. These technologies can facilitate deeper understanding and provide valuable insights, including:
- Suggestions for design and process optimizations
- Ideas for plant maintenance and control improvements
- Insights on operations instructions and training protocols
- Recommendations for incident assistance and resolutions
Virtual and augmented reality technologies empower manufacturers in the automotive industry with critical knowledge, clearly and visually, to improve the design and manufacturing process, as well as maintenance and training.
Example: Ford has implemented a virtual reality training tool that can teach technicians how to service and maintain all-electric Mustangs without having to physically access the vehicle model. “This virtual reality training tool allows technicians to understand the components and steps required to service the high-voltage systems, and then confidently perform diagnostics and maintenance.” – Dave Johnson, Director of Ford Service Engineering Operations
Advances in virtual replicas – digital twins
Since the dawn of Industry 4.0, digital twins have been growing in importance for manufacturers. Worth $4 billion in 2019, the digital twin industry is expected to reach $36 billion by 2025, reflecting a CAGR of 38%.
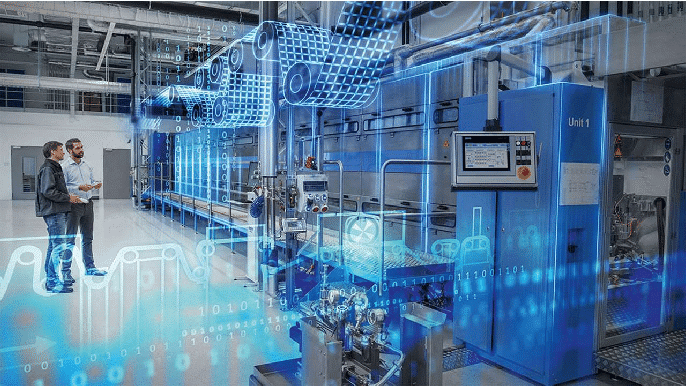
Digital twins use advanced machine learning algorithms to generate an accurate 3D model. In the automotive industry, digital twins can be a virtual replica of an entire component, a whole vehicle, or sections of a production line – complete with all of the electrical, mechanical, and physical attributes. Digital twins can benefit automotive manufacturers by:
- Providing a virtual model that integrates data from various sources. For example, combining performance data from a working component with historical performance data can facilitate design improvements.
- Predicting equipment malfunctions. A virtual model can enable engineers to visualize potential machine downtimes before they happen, ensuring continuous product throughput and maximum production efficiency.
- Accelerating the verification process of new features or designs. A digital twin can produce all the data needed to run simulations that provide accurate results, thereby eliminating the time it takes for engineers to verify the feasibility of their designs.
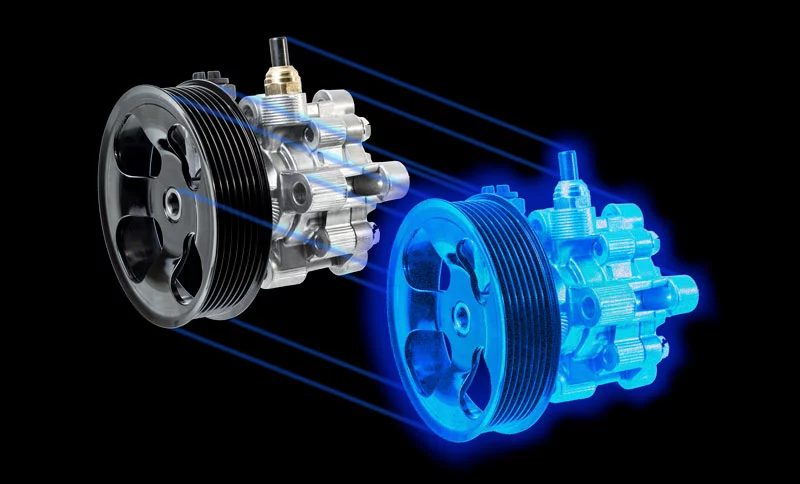
Example: A digital twin can provide a virtual replica of a vehicle’s hydraulic pump. Empowered with this digital twin, an automotive engineer can observe a precise virtual 3D model of the pump and manipulate it to see it from all angles, enabling them to identify any design flaws before it goes into mass production.
Human-machine collaboration
- Higher employee efficiency, as cobots reduce inactive time on the line and enable the performance of highly repetitive tasks without mistakes
- Improved manufacturing efficiency and reduced labor costs, resulting in a healthier bottom line
- Safer working conditions, as cobots can perform tasks unsafe for humans and in dangerous environments
- Advanced automation on the factory floor, as the progressive technology in cobots ensures continuous improvement on their ability to work with humans
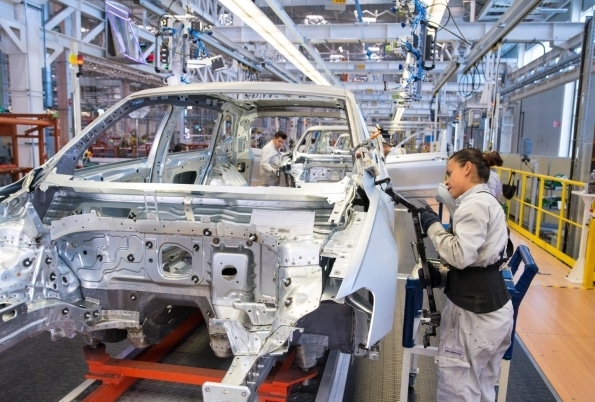
Example: Volkswagen has recently introduced cobots to their assembly plants in Mexico to support its workforce, to measure for gap precision, and help with final inspection checks. In the Puebla plant, a worker assisted by a cobot can accurately measure 26 checkpoints in less than a minute.
Additive manufacturing
Additive manufacturing has set new standards in production, including instant prototyping and 3D printing of a finished product. Automotive engineers can reap multiple benefits from additive manufacturing, including:
- Reduced scrap and material waste, since material is added as needed to create a product, as opposed to traditional methods, like milling or turning, which cut away and waste material
- Simplified production processes and product assembly, as additive manufacturing integrates the assembly process into one part. Traditional prototyping methods are more complicated and require more resources and multiple production steps.
- Lower prototype production times and costs compared to older technologies, like milling, which involves considerable configuring. Additive manufacturing is more efficient since prototypes can be produced and tested quicker and improvements made instantly.
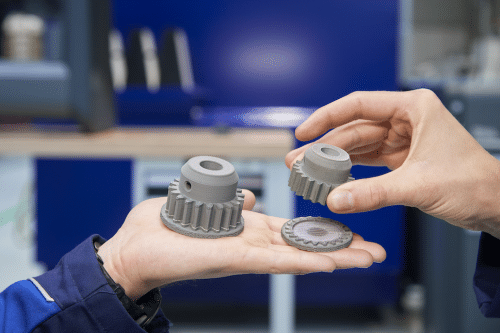
Example: BMW has invested €15 million in an additive manufacturing facility to bring their prototype and series parts production together under one roof. They are researching new 3D printing technologies with the objective of industrializing 3D printing methods to streamline their component manufacturing and speed up development.
Ready to adopt Industry 4.0 technologies in your enterprise?
Improved business outcomes should be the end goal of any new technology implementation strategy. Industry 4.0 has led to greater production flexibility, precision, and efficiency in the automotive industry. But the best solutions will not lose sight of the fact that it all comes down to the part being manufactured.
LinePulse is a predictive quality solution that helps automotive manufacturers reduce scrap and rework, and automate root cause analysis. We recommend adopting a predictive quality solution as an early investment in Industry 4.0 adoption. LinePulse is able to provide ROI right away. It is a scalable solution that can be implemented in almost any manufacturing environment, regardless of the existing tooling.
Share on social: