Statistical process control vs. machine learning
Last updated on March 15th, 2024
Most manufacturing plants implement Statistical Process Control (SPC) to monitor and manage their processes effectively. However, despite recognizing the potential benefits of advanced statistical methods like machine learning, many engineers at these facilities remain skeptical. Their reluctance often stems from a lack of understanding of the underlying data science.
In this blog, we aim to demystify both concepts for you. We’ll start with SPC, a familiar term in the manufacturing sector, and then introduce machine learning, a potentially new and valuable area for you to explore. While it’s clear that we advocate for machine learning solutions—given our expertise in offering machine learning services that enhance part quality for precision manufacturers—we believe in informed decision-making and hope to guide you through these innovative technologies.
What is Statistical Process Control (SPC)?
If you’re reading this article, you probably already have a solid background on Statistical Process Control (SPC), but here’s a refresher just in case. SPC is an efficient statistical method for monitoring and controlling manufacturing processes. It involves collecting and analyzing statistical data to ensure that a manufacturing process stays within pre-defined “control” limits.
Manufacturers employ SPC to scrutinize the processes of key characteristics that must remain within specific thresholds. The objective is to detect any statistical fluctuations through a well-defined protocol of sampling quantity and frequency, informed by a detailed capability or process study. By monitoring this data—particularly through tools like X-bar and control charts—operators can identify and address any signs of statistical variance, ensuring the process maintains its expected quality and consistency.
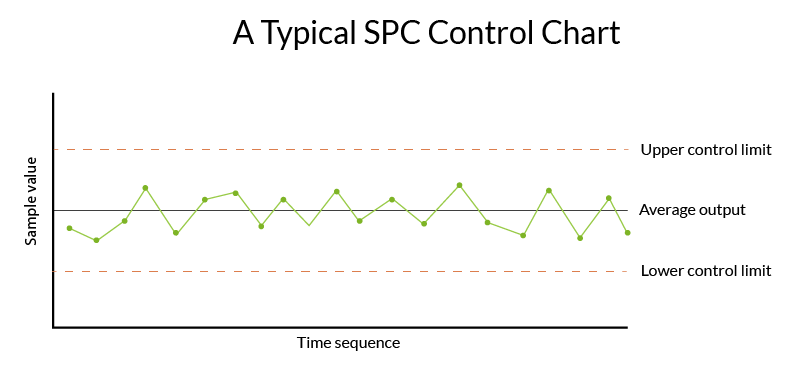
The limitations of statistical process control
SPC is an integral part of data analysis in most manufacturing processes. However, SPC has limitations, particularly in offering insights about overall process trends, or reducing defects in highly complex processes.
Even when a manufacturer has dozens of SPC charts showing good statistical control of in-process data, they still need to run end-of-line (EOL) tests. The fact that every pump, torque converter, transmission, and other part requires an EOL test to ensure its quality is a tell-tale that in-process monitoring through SPC control charts alone can’t ensure perfect quality.
SPC has limits in terms of what it can detect, predict, or analyze. These limits are exactly where advanced statistical methods like machine learning can fill in the gaps.
It is possible to derive advanced insights from a manufacturing environment that relies on SPC… but it requires a lot of manual effort. Usually, SPC data is siloed at each operation in the manufacturing process, which means that it needs to be collected and combined from multiple locations to understand the process in its entirety. Then, someone with a good understand of data needs to combine, sort, and analyze it. Using standard tools, this process can be immensely time-consuming. It requires one person to have both a solid understanding of the manufacturing process, and of data analysis techniques. That’s like doing two very different jobs in one.
At some facilities with processes that are not highly automated or that use older machines, statistical process data is generated manually, with operators filling in charts or spreadsheets by hand. This can data can prove even more challenging to gain insights from, since it may contain many errors.
How is machine learning used in manufacturing?
Machine learning, a subset of artificial intelligence (AI), enables computers to process, reason, and make statistical decisions based on data. This AI facet uniquely learns and adapts to new information autonomously, without needing human guidance.
In the realm of manufacturing, where vast amounts of data are routinely collected, much of this valuable resource remains underutilized. Machine learning steps in to analyze large, complex datasets at speeds unattainable by human effort, unlocking data insights that can significantly enhance operational efficiency.
There are two basic ways that machine learning can be used to analyze process data in manufacturing.
1. Analyzing data after collection
Custom machine learning models can be built and deployed to analyze data after it has been collected from various sources on the manufacturing line.
These models can be built by an in-house data science team, or by external machine learning experts and consultants. They require data science and machine learning experts to collaborate with engineers to ensure that the right data is used for the right purposes.
These types of solutions are preferred when there is a single manufacturing problem to be solved, and the problem does not require an urgent solution.
2. Analyzing data in real time
Machine learning software platforms now exist that can ingest manufacturing data in real time. An example of this is a predictive quality platform. These solutions contain machine learning models and algorithms that have been purpose-built for the manufacturing environment. They are scalable, which means they can be applied to solve a wide range of problems on the shop floor, and even adapt when specs change or retooling occurs on the line.
Real-time platforms are configured when they are initially set up, so that the platform understands the unique setup of each manufacturer’s lines and process. This can include things like operation order and names, model types, etc. Once they are configured, the platforms ingest data constantly, meaning they always have organized data on to be analyzed instantly when a problem arises.
Real-time machine learning platforms are preferred for manufacturers who want to be ready to solve any problem that occurs on the shop floor. They can be especially useful when setting up net new lines or launching new products, since they help users understand the relationships between different aspects of their process.
Machine learning supplements traditional Statistical Process Control
Machine learning and statistical process control (SPC) are not mutually exclusive. In fact, machine learning has been proposed as a method for augmenting the construction and interpretation of SPC charts, and we couldn’t agree more.
Machine learning is a valuable complement to Statistical Process Control (SPC) checks, not a replacement. It addresses questions such as, “Why do my parts fail the End-Of-Line (EOL) tests even when they meet specification limits?” While it might seem feasible to apply SPC to EOL test data, machine learning offers deeper insights by analyzing interrelations within the data.
Unlike SPC, which typically monitors single data trends to anticipate nearing control limits, machine learning examines multiple data streams concurrently. It leverages its computational strength to uncover connections within and between these data streams.
Consider a transmission’s EOL test, generating over 500,000 time series data points. Machine learning excels by discerning patterns and anomalies among these points and correlating them with data from similar tests. This capability to detect interrelated patterns far surpasses what traditional tools or manual analyses could achieve.
Imagine the impracticality of an individual trying to sift through half a million data points to decipher relationships and draw conclusions. Machine learning streamlines this daunting task, enhancing both efficiency and accuracy in data interpretation.
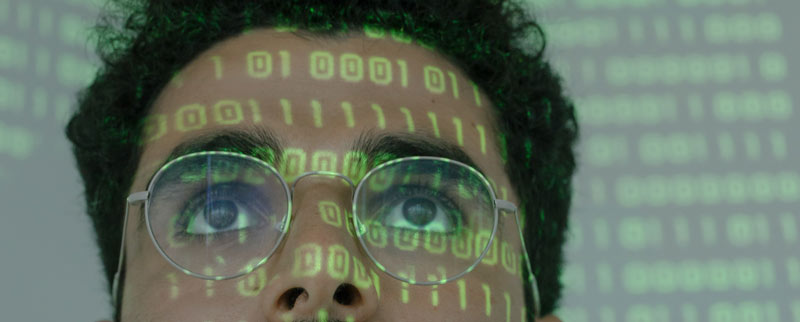
Even if you apply statistical process control techniques to monitor key machine signals (after determining which signals are crucial), you would only detect trends relative to your control limits. However, this doesn’t necessarily indicate a defective unit.
Machine learning delves deeper by examining the interrelations among various signals, unlike SPC, which merely tracks the trend of individual signals over time. This comprehensive analysis is what sets machine learning apart, offering actionable insights beyond the mere identification of trends. While SPC can alert you to a process trending towards or away from set parameters, it doesn’t provide solutions or actions to rectify the situation.
The fixed control limits of SPC are essential for some manufacturing scenarios. For example, there’s a particular part feature influencing downstream assembly that doesn’t require constant monitoring according to a capability study. If the process capability for this feature is adequate (with a Cpk between 1.0 and 2.0), you can sample the data and use SPC to keep this feature within control limits, preventing downstream assembly problems.
On the other hand, machine learning offers a more intricate understanding of your entire manufacturing process. It can detect hard-to-spot trends in machine signal data to warn engineers and operators of problematic trends that could lead to quality issues, so they can act quickly to prevent them. It can also provide invaluable statistical information about the potential causes of a defect, which can accelerate root cause analysis.
The future of Statistical Process Control
We think of machine learning as a progression from statistical process control. They are both methods of data science, after all.
Machine learning is the logical next step in response to the growing volume of manufacturing data that most plants generate. In the same way that the invention of the Internet built upon and expanded the capabilities of the computer, machine learning adds exponential possibilities to draw connections, detect patterns, and connect seemingly disparate data together to form a whole picture of your production.
The development of real-time machine learning platforms present the next generation of manufacturing analysis, and with their expanded capabilities, create a new category of quality management that some refer to as “Quality 4.0”. We predict that manufacturers who don’t embrace machine learning will eventually be left behind, as their competitors embrace this new technology and reap the benefits of increased productivity, efficiency, and reduction in waste.
If you’re still new to the topic, don’t worry. There is still plenty of time to learn about machine learning and how to adopt it in your manufacturing environment.
Do you need to add machine learning to your SPC strategy?
SPC can be effective when you’re analyzing single-variable data within a normal distribution. However, when facing complex, multivariate data, machine learning becomes a vital tool. If your manufacturing process appears to be on target according to statistical process control charts, yet you encounter unexpected issues in subsequent assembly stages, it may be beneficial to integrate machine learning with your existing statistical methods.
Plants that struggle with consistently high scrap and rework rates may also benefit from incorporating machine learning. Poor quality metrics can often indicate a process that is not thoroughly understood, and machine learning algorithms can be used to reveal dependencies and oversights that can help optimize it.
Most manufacturing environments today already generate substantial data sets, setting the stage for effective machine learning application. Some manufacturers are generating substantial amounts of data and paying a high price to store it, but do not yet have the tools to get the most value out of it.
Every facility must evaluate the cost of their quality issues and determine whether they are collecting the right data to gain useful insights from a machine learning project or product. Consulting with experts in both data science and manufacturing can help determine whether they are in the right position to implement machine learning.