Predictive quality is a better investment than predictive maintenance
Last updated on June 19th, 2023
Developing an Industry 4.0 roadmap is critical for moving manufacturing facilities into the modern age and helping them stay competitive in today’s marketplace. An Industry 4.0 roadmap should consider all possible investments that could make an impact on a site’s specific production environment, and prioritize the investments according to their potential effectiveness. The roadmap also needs to be an evolving document that can consider new technologies as they gain maturity.
Before we explore some of the content that should be in your facility’s Industry 4.0 roadmap – a quick note about us. Acerta was founded on the premise that machine learning and artificial intelligence can improve operations in automotive manufacturing, and address some of the biggest problems the industry faces: meeting consumer expectations for quality, staying ahead of competitors in terms of quality issues, and preventing quality-related warranty issues or recalls.
To ensure that precision manufacturers can meet their quality targets and produce high-quality parts correctly the first time, we developed LinePulse, a predictive quality solution that reduces scrap and rework, improves first time through, and increases operational efficiency. Our solution is often compared to the effects of another category of tools, which are predictive maintenance solutions. It is important to understand the differences between predictive quality analytics and predictive maintenance, to ensure the right one is on your roadmap.
Let’s explore what each solution is, its benefits and drawbacks, and why we recommend a predictive quality solution as a higher priority investment in most cases.
What is predictive maintenance in manufacturing?
Predictive maintenance refers to methods that use condition-monitoring tools to monitor performance and activity of structures or pieces of manufacturing equipment while they are operating. This data is analyzed and used to identify when machines are wearing down and nearing failure.
Many different tools and systems can be used in this process. For the purpose of this discussion, we will assume the most advanced form of predictive maintenance is being used. This type of predictive maintenance uses machine learning and artificial intelligence to analyze and model the data. These models can generate the most accurate predictions available today, and require less manual effort to administer than other methods.
It is also important to understand the precursors and alternatives to predictive maintenance that can be used in manufacturing facilities.
Three methods to control maintenance in manufacturing
There are three methods of handling equipment maintenance in a manufacturing facility, predictive maintenance being one of them. Understanding all three of these methods provides a short history of controlling machine maintenance in manufacturing, as well as allowing us to compare the possible options available to manufacturers today.
1. Corrective maintenance
A corrective maintenance approach waits for machinery to break down before initiating repair. A benefit of this method is that machinery and its parts are allowed to live out their full lifespan, which can reduce the cost of replacing parts.
A downside to this approach is having to deal with unexpected, and sometimes prolonged, equipment downtime. A breakdown could happen any time, such as in the middle of a critical production period.
Additionally, repairs with this method can be costly. A full machine failure can sometimes be the result of a domino effect of smaller, more inexpensive parts inside the machine breaking down until they finally affect a larger, more critical and more expensive part.
2. Preventative maintenance
Preventative maintenance uses a fixed schedule to make repairs and maintain manufacturing machines. A plan or maintenance schedule is put into place, using information about typical machine’s lifespan and usage. The schedule may indicate that a part should be replaced every 3 months, or after every 20,000 cycles.
This method can help to plan machine downtime, making it easier to avoid impacts on production. Machines can be serviced in off-hours or during slower periods. Maintenance events tend to be easily quantifiable, and costs of the parts are predictable.
The downside to this method is that a schedule can only estimate the true life of a part but there is a chance it could wear out sooner than predicted. More likely, the part will get replaced before it reaches the true end of its life, which drives up the cost of spare parts and the cost to perform the maintenance.
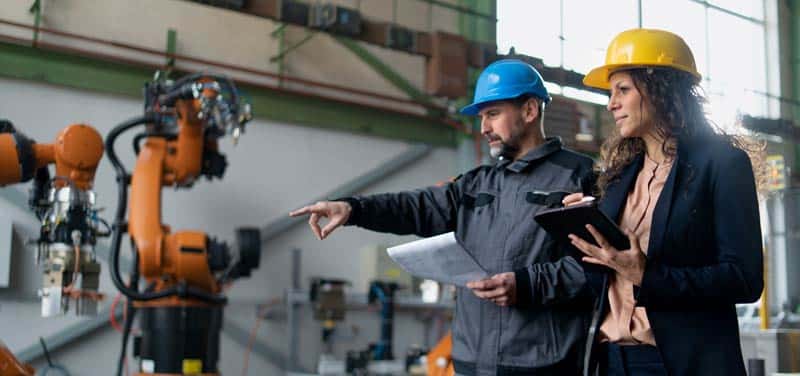
3. Predictive maintenance
Predictive maintenance is the act of monitoring machine health through data. This data can be monitored actively, and with machine learning and AI tools, very accurate predictions can be made about when machines and their composite parts are nearing their end of life.
This method provides the best of both worlds. It maximizes the lifespan of parts, meaning that it has the lowest possible cost for spare parts. Since it helps to forecast the type of maintenance events that are about to be needed, labour and costs can be calculated in advance and scheduled at convenient times. Predictive maintenance can also increase workplace safety by avoiding destructive failures.
What is predictive quality in manufacturing?
A predictive quality solution is similar conceptually to a predictive maintenance one in that it uses data, analyzes it, and models it to make predictions. In the case of quality, the data inputs come from the signals that manufacturing machines and devices generate, such as speed, temperature, torque, location, size, and anything that can be tracked. This data is monitored and can be traced against serialized parts so that each part has its own data history. Machine learning and AI can then predict when defects will occur based on patterns in the data, and ML/AI can help identify the root cause of a failure.
Three methods to control quality in manufacturing
The methods to manage quality in manufacturing are also comparable to the three methods of equipment maintenance described above. Let’s explore the pros and cons for each method.
1. Corrective quality management
A corrective (and reactive) approach to quality is the “old fashioned” way of managing quality, but it is still very prevalent in many manufacturing facilities. Corrective quality is the act of taking a defective part and either reworking it into one that meets the quality specifications or scrapping it.
Scrap and rework can sometimes be thought of as a lower-cost option (compared to a preventative approach), since the extra resources required are only applied to the parts that are in fact defective.
However, in many cases such as injection molding processes, rework is simply not possible. The work cannot be redone, and the defective part must be scrapped. This can make it very costly and inefficient.
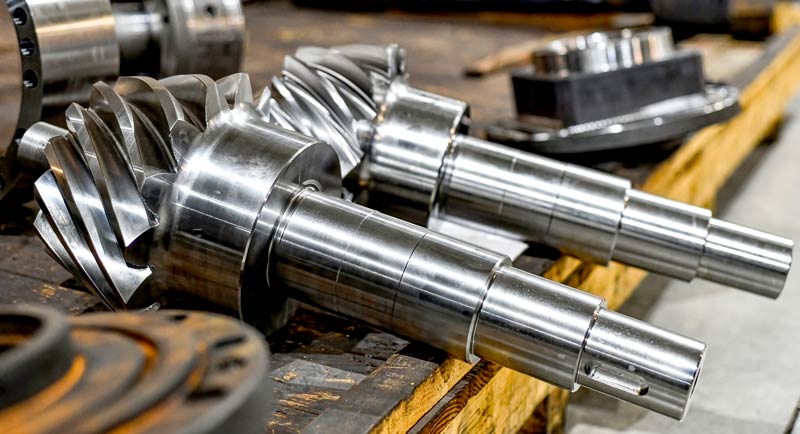
2. Preventative quality management
Preventative quality management methods include a variety of techniques that can be applied together or in isolation. They focus on process improvements, and tightly monitoring the progress of parts as they are built. The most common techniques are:
- Process design and control
- Tightening specification limits
- In-process verification
- End-of-line testing
These methods can sometimes help to prevent quality issues, or they can prevent a part from getting too far through the production process before an issue is detected. However, since they lack focus in solving specific quality issues, efforts may be wasted in areas that are not problematic. Another downside is that these methods are often costly to implement and often require large infrastructure changes.
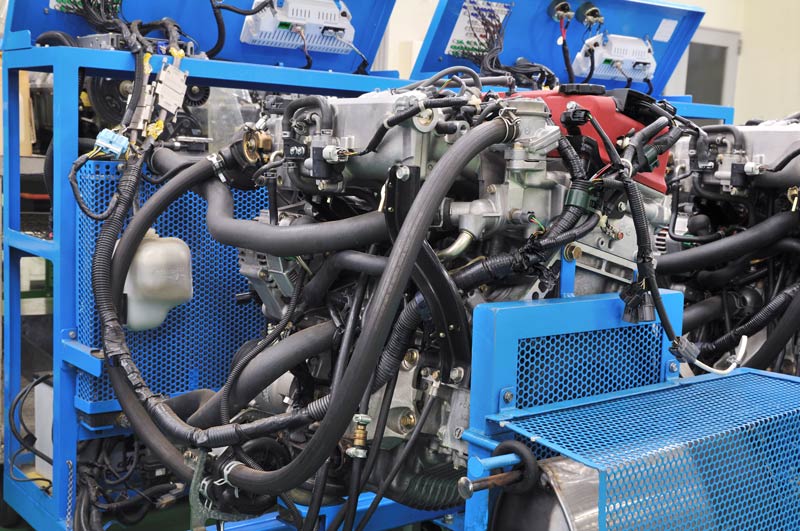
3. Predictive quality
Predictive quality management addresses specific issues on the manufacturing floor by taking operational and part data from machine signals and using them to make predictions about part quality. It does this through machine learning and artificial intelligence algorithms. Through advanced analytics, it can predict when defects will occur and help to identify the root causes of those defects in real time. This allows quality and process engineers to make adjustments and implement solutions before possible quality issues impact production.
Predictive quality management software learns about the production cycle from monitoring patterns in a facility’s data. It becomes extremely customized to the production environment, which makes it targeted and strategic. Because of this, the investment is much more likely to have an impact on revenue than many other preventative measures.
In addition, relying on a predictive quality solution can save time in production, as there is less reliance on lengthy processes such as end-of-line testing. The technology can also be applied to end-of-line testing to make it faster and more efficient. This is one of several ways that predictive quality management can increase operational efficiency in a precision manufacturing plant.
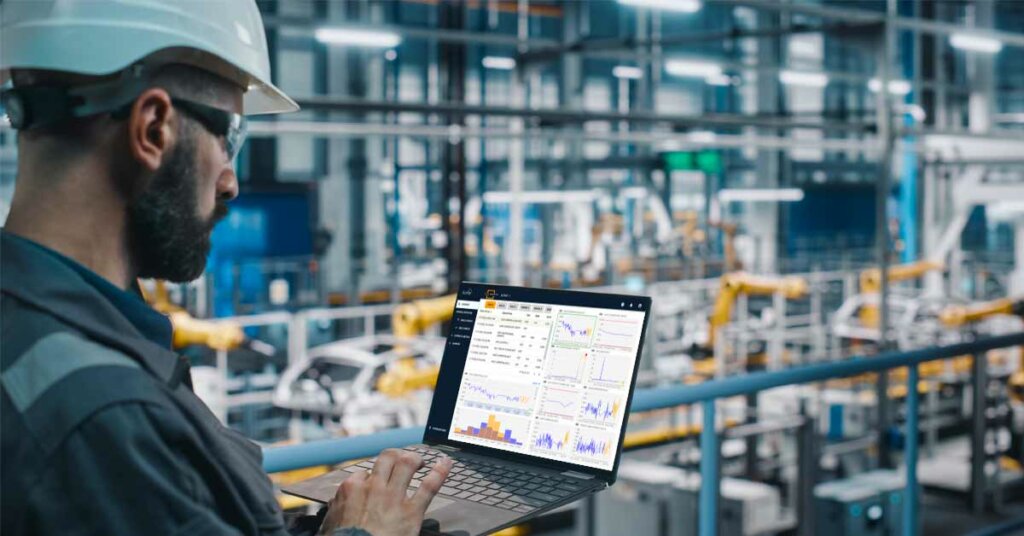
Benefits of a predictive maintenance solution
If an investment in only one Industry 4.0 technology can be made, it is important to compare these two types of technologies. Relying on a predictive maintenance solution as the main method to control production quality has one main benefit.
Machine uptime and OEE are easy to measure
Overall Equipment Efficiency (OEE) is a discrete calculation in which machine uptime and performance are prioritized. The impacts of a functioning machine can be demonstrated by anyone with a calculator. If you know how many parts per hour are produced and how much revenue they generate, then you can assign a direct cost to each hour of downtime.
Plant managers who rely on these clear-cut numbers only may be partial to implementing a predictive maintenance solution, as they can easily show the ROI.
A predictive quality solution can be harder to measure. An easy calculation to look at is first time through. If this improves, it is clear that quality is improving.
However, improving part quality often has larger repercussions. Defects that are not caught in the facility and passed on to a customer can have an extremely large financial and reputational cost. Using a predictive quality solution drastically reduces risk in this area. But since these events are so random, how can we attribute a dollar value to this reduction in risk? How can we attribute a dollar value to reputational damage? The part quality equation is more complex.
The challenges of a predictive maintenance solution
Unfortunately, predictive maintenance is not the optimal solution to ensure that quality is optimized during production. Here is the downside of relying on it as the primary driver to improve quality.
Predictive maintenance affects process quality, not part quality
Focusing on fixing a process has been the main method of controlling quality for the greater part of the 20th century. There is no doubt that well-functioning machines can produce better parts than broken ones. However, today’s process optimizations are already quite streamlined and accurate, as they have been built upon so many decades of refinement in the industry.
Today’s quality defects are less likely to be caused directly by a failing machine, and more likely to be a result of small miscalibrations, or a combination of seemingly random events from well-functioning machines. We have designed our processes so well that the amount of process improvements available to us tend to be minimal. This is why a focus on the part itself is essential to take the next step in ensuring quality. Fixing a process will not fix a part with poor quality.
Where should you invest?
If your facility is faced with a choice between two investments — a predictive maintenance solution and a predictive quality solution — we believe a predictive quality solution will have a stronger impact on your overall production and revenue. Here’s why.
1. Predictive quality gives a higher ROI
The main goal of a manufacturing facility is to produce and ship quality parts. It isn’t just to keep the machines in good working condition. By focusing resources on what is causing defective parts to be produced and why, the real issues can be identified quickly and part quality can be increased. Scrap and rework can be reduced.
These efficiencies translate directly into increased revenue.
Predictive part quality solutions can also reduce the need to rely on in-process verification or end-of line testing. These types of tests require expensive specialized machinery to be installed, and in many cases require extra labour costs to administer. This is another area of savings.
Lastly, by preventing downstream quality issues that affect customers, there are untold savings on warranty issues and their resulting reputational impacts.
2. Predictive quality can indirectly affect maintenance
Though it is not designed to focus on manufacturing machine maintenance, the predictive quality solution can indirectly help to identify signs that point to machines wearing down or approaching a breaking point. If there are warning signs that defects are in danger of occurring, the tool can use automated root cause analysis to determine which stations or signals are related to the problem. This can highlight equipment that needs investigation. The problem could be related to maintenance, which can help to determine if a piece of equipment needs fixing.
If your facility can already effectively run a production line with moderately high equipment uptime and performance without an AI-powered maintenance solution in place, then the predictive quality solution will offer more overall value.
3. Predictive quality solutions can scale quickly and easily
Predictive quality solutions can learn quickly about the production environment and begin to predict issues sooner, since the volume of data regarding defects is much higher than the amount of data available about machine failures. When new signals are added to a line, they can be added immediately to the predictive quality tool. New lines can also be added quickly and easily.
As robotics and manufacturing equipment become more advanced, they tend to collect more and more data. The precision and effectiveness of predictive quality solutions will only continue to scale as the data collected improves, which further decreases the need for preventative measures such as in-process verification.
Predictive quality solutions are scalable not only across multiple lines or production environments, but also over time, as more and better data will only improve the results they can provide.
Share on social: