Last updated on December 11th, 2023
Case Study
Diagnosing engine failure modes with AI
Objectives
- Improve vehicle diagnostics by predicting failures in five different engine platforms
- Identify the causes of engine failures using test signal data
Challenge
- Client data collected from four engine platforms, each of which had 4-5 different test profiles
- Client required accuracy of 80% or better for failure prediction
Key Results
93%
Accuracy for failure prediction
100%
Identified suspect signals in engine test signal data
Background
A leading Tier-1 engine supplier needed to improve its vehicle servicing and diagnostics to reduce the rate of breakdowns and accelerate maintenance and repairs. The company had been relying on aggregated diagnostic trouble codes (DTCs) collected via the OBD port to indicate when vehicles needed to be serviced, but its existing diagnostic tools were unable to capture four distinct engine failure modes. Moreover, investigating the source of these failures was difficult and time-consuming.
The Problem
The client needed a solution that could predict failures for four different engine platforms. The signal data from each engine was generated through 4-5 different test profiles. In addition, the client required a platform that could predict engine failures with an accuracy rate of at least 80%. Moreover, because the client’s goal was to improve its diagnostic tools, the platform’s predictions needed to be delivered in real time.
Solution Process
Acerta began by exploring the client’s sample data—analyzing the signals and evaluating the viability of the machine learning models utilized by our Advanced Anomaly Detection module. A fundamental question faced by our data scientists was whether to use one model for all four engine platforms or one model per platform. In the end, we elected to use different models for different platforms, as well as for different stages in testing. The client’s two goals also required two different machine learning approaches: classification as well as anomaly detection.
This led to Acerta’s data engineers building a nested data pipeline for the classification and anomaly region results which delivered them to the client’s engineers in real time. The LinePulse Advanced Anomaly Detection dashboard displayed the engine id, the name of the data trace, whether the unit was passing or failing, an abnormality score, and highlights of any anomalous regions in the signal data.
Results
Acerta’s models exceeded the client’s requirements for accuracy in predicting engine failures at a rate of 93.3%.
Furthermore, the LinePulse Advanced Anomaly Detection module successfully identified suspect signals and anomalous regions within the engine test signal data, enabling technicians to catch more failures and reduce the time it takes to investigate them.
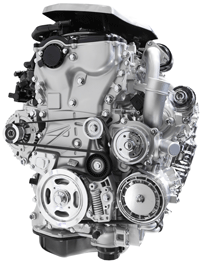