Is Six Sigma still relevant in industry 4.0?
Last updated on June 19th, 2023
Six sigma was created by Bill Smith in 1986 at Motorola. The concept was intended to improve manufacturing quality by removing the causes of defects in manufacturing processes to an even greater extent than it had in the past.
The term “Six Sigma” originated from statistical modeling of manufacturing processes. Sigma, or “σ,” is a Greek symbol that is used to measure deviation from the mean. Why six? “Six Sigma” represents a normal distribution, in which there are six standard deviations (represented by “σ”) away from the mean and each specification limit.
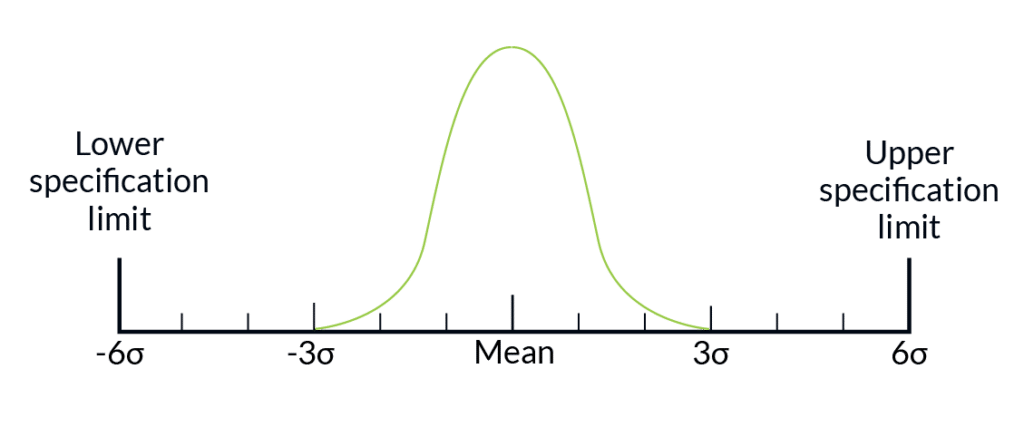
Later, this quality target was raised slightly to 3.4 defects per million units — still a very high standard of quality to aim for. Prior to Bill Smith’s work at Motorola, it was common to measure manufacturing defects in thousands rather than millions. This earlier standard was based on a normal distribution in which specification limits were three standard deviations from the mean instead of six.
Motorola achieved an increase in quality through meticulous application of statistical control methods through the manufacturing process and product specifications. Eventually, as Six Sigma became a more formalized approach, a method of continuous improvement or DMAIC (define, measure, analyze, improve, control) was also added to the playbook.
Contributions to Six Sigma from automotive manufacturing
The pioneer of quality and standardization, Henry Ford
Until Six Sigma came about in the 1980s, great advances in quality control and quality assurance had already been made. When the Ford Motor Company introduced the assembly line in the early 1900s, they also introduced effort to control quality from the very design of the process.
Standardization of parts became necessary, as individual stations were isolated from each other with the promise that by adhering to specifications, everything would fit together and function properly at the end.
Prior to this style of manufacturing, products were largely produced individually and by hand. An individual or small team would create each part of a product and assemble it, fitting individual pieces together to produce similar – but not identical — products.
The impact of Ford’s assembly lines to the manufacturing industry cannot be overstated and this method of manufacturing completely changed how we think about quality. Without this concept of standardized parts, which were later managed with Statistical Process Control, Six Sigma would not exist.
Toyota and Japanese manufacturing contributions to Six Sigma
In the 1950s and 1960s, Japanese manufacturers made great strides to improve the efficiency and output of their factories. Toyota was particularly known for its innovative developments. Some core concepts from Japanese automotive manufacturers during this period that made their way into Six Sigma are:
- Kaizen – meaning “continuous improvement”, or a way of improving business processes and methods loosely comparable to the scientific method
- Total Quality Management – organizational efforts to improve quality across all departments of an organization, not just production
- Kanban Squares – the alignment of inventory levels with real product consumption
- Quality Circles – groups of workers performing the same job who get together regularly to identify, analyze, and solve problems related to their roles.
- Just-In-Time manufacturing – A production method to reduce time by focusing on matching production to demand
The Six Sigma principles
Six Sigma is comprised of a collection of core principles and two process methodologies. The principles include:
- Undergoing continuous efforts to achieve predictable output from processes will result in business success.
- Continuous process improvements should increase benefit to the customer as well as financial return for the organization.
- The entire organization must be committed to achieve sustainable quality improvement.
The two process methodologies used in six Sigma are called DMAIC (used for existing projects) and DMADV (used for new projects).
DMAIC (Define, measure, analyze, improve, control)
- Define the system, project requirements, and goals.
- Measure aspects of the current process, collect data, and measure the current process capability.
- Analyze the data, determine what the relationships are between it, and find the root cause of defects.
- Improve the current process based on the data analysis to create a new future state.
- Control the future state process to ensure that errors are corrected and defects are avoided. Implement control systems to monitor the process and repeat until the desired quality level is obtained.

DMADV (Define, measure, analyze, design, verify)
- Define goals that align with consumer demand and company strategy.
- Measure aspects that are critical to quality product capabilities and the state of the production process.
- Analyze and design alternative process methods.
- Design improved alternative process based on the previous analysis.
- Verify the design with pilot runs, implement the new process and hand it to the process owner.
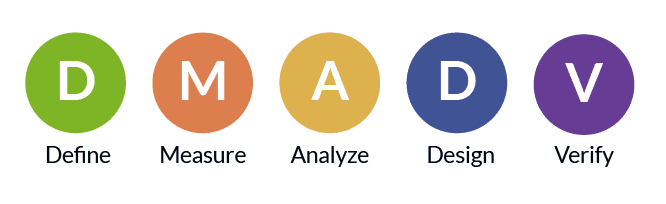
Six Sigma in manufacturing today
Six Sigma is widely used across the automotive manufacturing industry. which is the ideal industry to benefit from Six Sigma quality improvements because the products produced are typically very complex and require a variety of manufacturing processes.
Automotive manufacturers also have a lot to lose if their products are not of sufficient quality. The infamous Takata airbag recall is a great example of the risks that automotive manufacturers face when producing defective products. The company faced bankruptcy after more than 122 million airbags were recalled from dozens of vehicle makes and models. The recall was estimated to cost over $24 billion and bankrupted the company.
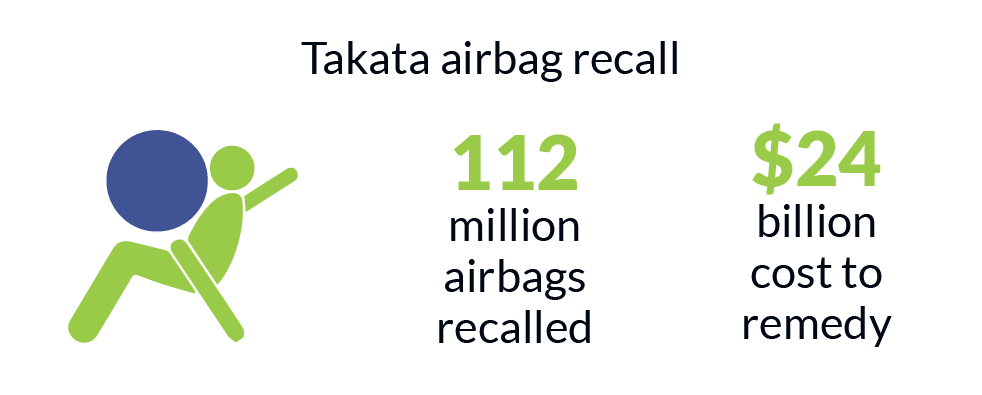
With these figures in mind, Tier-1 suppliers and OEMs take quality incredibly seriously and devote significant investment and labor towards perfecting their products. Since 6 Sigma represents the culmination of a century’s worth of research on quality management in manufacturing, it is this methodology that continues to dominate the conversation in quality.
Does Six Sigma hold up in Industry 4.0 and the future of manufacturing?
As industry 4.0 marches on and cutting–edge innovations become commonplace in manufacturing facilities, the next generation of tools and technologies are beginning to emerge.
Will Statistical Process Control evolve beyond traditional control charts?
The concepts of Six Sigma were traditionally applied to manufacturing in an environment where statistical process control was the most advanced method available. Today, statistical processes performed by a Six Sigma black belt are much more complex than the typical control chart, but are too complex to be applied in every scenario. The control chart has continued to dominate quality control due to its simplicity and wide applicability.
Artificial intelligence also allows the general population to access much more advanced analytics than they would have the time and resources available for in the past. The use of artificial intelligence and machine learning to monitor signals and perform complex analytics quickly has allowed manufacturers to intervene in the process earlier to prevent potential defects down the line.
New methods of analysis that compare multiple variables and warn of potential quality issues use SPC concepts as inputs, but are significantly different and more powerful in identifying areas where the manufacturing process breaks down.
The shift towards accessible advanced statistical processes is becoming more available with machine learning and artificial intelligence and begs the question of the basic control chart’s role at the core of quality methodologies. As artificial intelligence makes advanced analytics more accessible, will there be a new “basic” model for measuring quality in production?
Will advanced robotics increase the standard of quality beyond 3.4 defects per million?
The use of robotics and automation in manufacturing has replaced more and more manual tasks. This has increased overall precision and accuracy in automated production. As machines and robots continue to take over more of the manufacturing process, the level of standardization across the entire assembly line improves.
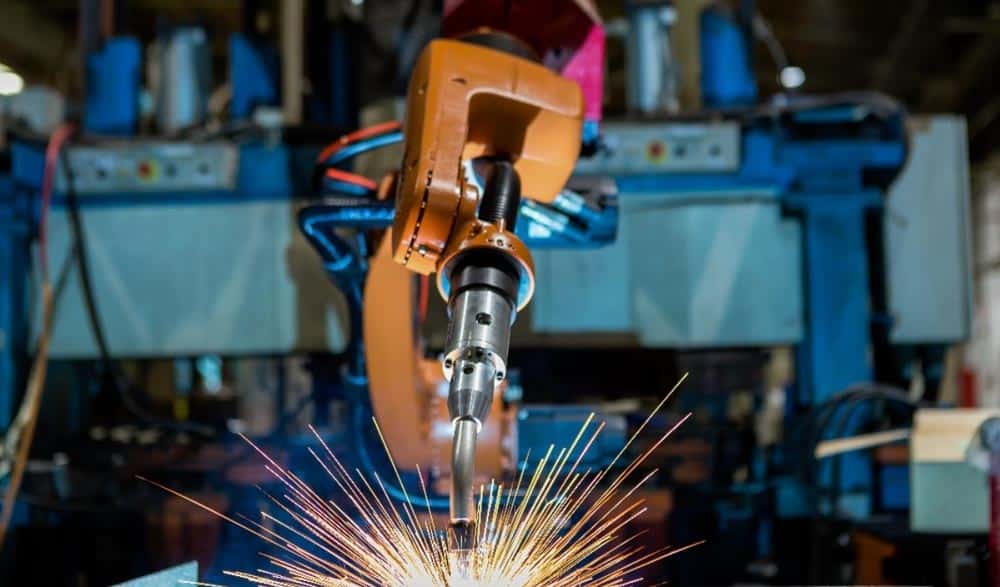
As the expectation of quality from production improves, will our quality targets get higher in the future? Will the industry be measuring quality in terms of defects per billion instead of defects per million? The jump from measuring defects per thousand has already occurred, leaving the imagination to speculate that it could increase again.
Some automotive manufacturers might be satisfied with 99.99% quality, and will focus further investments on how to maintain that standard, but in a more affordable way. In some manufacturing sectors however, the push towards overcoming that last 0.01% is worthwhile. For medical device and aerospace sectors for example, 3.4 defects per million is already too many.
Could Six Sigma’s linear process methodologies evolve to agile in manufacturing?
The “define, analyze, design, verify” life cycle of a project looks very similar to what came to be known as the waterfall style of project management in the 1970s.
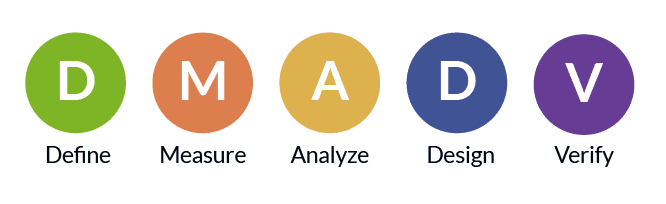
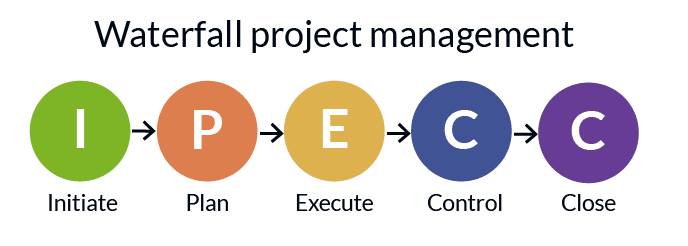
Although the steps are labeled differently, both processes begin with defining or initiating the problem or challenge, and include some aspect of planning, measurement and analysis followed by design and execution. Both also include testing and verification. Each step in the process must be completed before the next one begins.
When software development began formalizing into more mature business practices, the concept of agile project management came about. Instead of a linear sequence of steps, agile was characterized by a cyclical process in which there are frequent feedback loops between the planning, execution, testing and feedback stages. Software was released in a series of iterative forms which were continuously improved as they were developed, and new features were added even after the product was released to customers.
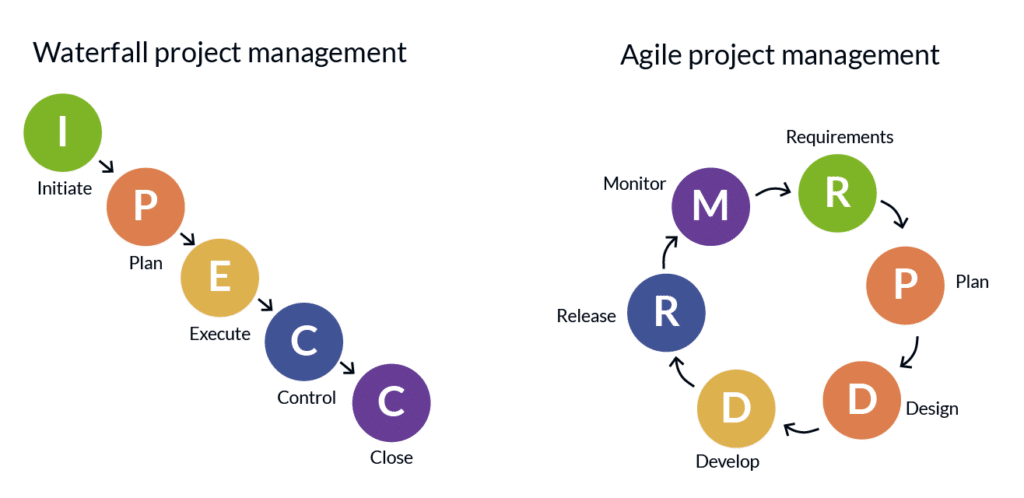
Perhaps a future development of the Six Sigma DMAV methodology in manufacturing could look like an agile equivalent. With tools such as advanced analysis from artificial intelligence, self-maintaining and self-adjusting robots, and faster development cycles, could manufacturing also see a continuously looping version of the design-measure-analyze-improve cycle?
Scaled agile framework (SAF), an adaptation of agile and lean business practices, has already been developed to apply these practices in large organizations. Agile manufacturing exists in principle, although applications are in their infancy. Agile works well with software: changes can be made with little cost and installation time since all the work is digital.
There are many obstacles in creating an agile manufacturing line such as the cost of new materials or changes, machine downtime, and time necessary to test new designs for safety and performance. But there are some ways that manufacturers are still moving in an agile direction. Digital twins have allowed manufacturers to model production optimizations and changes in their manufacturing processes to ensure that they can be implemented quickly with little risk. They can test and perfect new processes and product designs digitally before making physical changes.
Applying agile to manufacturing doesn’t necessarily mean making dynamic changes in the design of the same part through production. It could also refer to a flexible manufacturing facility or “flex line” that adjusts to meet the needs and requirements of different products as new information comes to light.
Will Industry 5.0 customization trends cause a shift away from uniform, identical products?
The above questions assume a continuation of Industry 4.0 trends into an inevitable future. As technology advances, everything will get faster, more accurate, more efficient, and more standardized. This evolution can be compared to the history of computer chips becoming smaller and smaller, beyond what humans once thought was possible.
However, many predictors of Industry 5.0 trends have indicated a change in direction from this continuous advancement towards perfect standardization and uniformity. A shift towards product personalization has been highlighted. It is also predicted that humans and robots will be co-working, which has the potential to bring back a pre-industrial “craftsman” style of producing products: where no two are exactly alike. If manufacturing were to shift more towards this style of producing custom, unique products, it is easy to see how Six Sigma would lose some amount of relevance. Six Sigma is built on the assumption that standardization and adherence to tight product specifications are what defines quality.
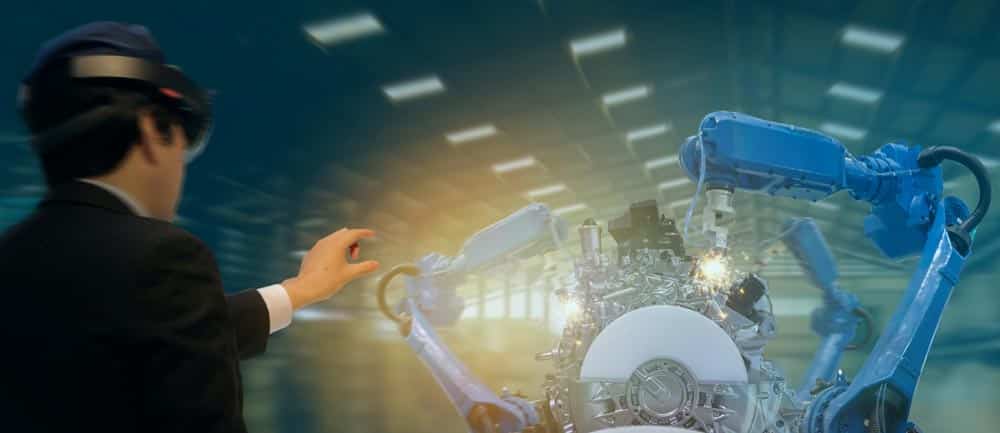
It is hard to picture an automotive manufacturer creating a vehicle with completely custom engine components or brakes. How would the vehicle function or pass safety tests? How would a part be replaced if something broke down? Certainly, there will always be a strong need for a set of standardized parts to serve functional requirements in manufacturing. Perhaps customization in automotive takes the form of an ever-increasing suite of trim options for vehicles. Final vehicles coming off an assembly line could look quite different from one another and be completely customized for each consumer, but the core parts will continue to have standardization between them.
Managing increasing levels of customization while assuring quality at the same time requires even more focused efforts on quality as vehicle construction becomes more complex. Artificial intelligence and advanced robotics will make this possible.
Six Sigma in the near future
Some of the thought experiments above may seem far-reaching or they might be realized too far in the future to consider in today’s Industry 4.0 landscape. It can be challenging to think ahead to such future changes and visualize what a well-established paradigm might possibly look like. But, if history has taught us anything, it’s that concepts and strategies regarding quality management will never stop evolving.
Members of the Acerta team with experience in both the automotive and technology sectors have lots of opinions so we asked them whether they thought Six Sigma is still relevant in Industry 4.0 and beyond.
Rob Plumridge, Product Manager
“Six Sigma is still relevant. The biggest danger in accepting innovative technology is to reject all traditional approaches and replace them with new tools. Traditional ideas have worked for a long time for a reason, and in many cases, it is possible for new and old ideas to coexist.
Six Sigma, for example, is a traditional methodology that is so widely accepted, it would have to take an incredible change for it to fade away. Industry 4.0 tools allow us to look at quality issues using non-traditional statistical methods, but they still support the overall goal of improving quality. Many Industry 4.0 tools still use Six Sigma concepts such as SPC as a foundation for their technology, but augment them and make them more accessible.”
Aaron Alberts, Senior Account Executive
“Six Sigma is, and will continue to be, relevant. In fact, the promise of Industry 4.0 for creating access to democratized, digital manufacturing data, could make Six Sigma even more effective. Six Sigma is not the only tool that manufacturers will have at their disposal, however. I see a future where AI and machine learning work constructively with Six Sigma, and will offer a new, data rich environment to give manufacturing professionals the clearest picture of what is happening in their ecosystem.”
Alan Tan, CTO
“Six Sigma is a great example of how IoT data can play an important role in manufacturing quality control. Six Sigma has become widely adopted because of its simplicity, its concept of controlling the allowed deviation from the operational norm, and because it uses a “canned” statistical approach compared to modern analytical methods. It is possible to calculate the metrics by hand, or using Excel.
However, as the amount of measurement data explodes, it is no longer sufficient to rely solely on early tools like Six Sigma to offer complete methods for quality management in the digitized manufacturing era. After decades of advancement in machine learning theory and practice, we are no longer confined by the same constraints around the amount of data, complexity of the calculation, and timeliness of training to perform more advanced analysis. Machine learning unlocks many possibilities with IoT data collection and machine learning models are highly capable of learning the operational norm by crunching data. They can either differentiate odd data patterns or predict the likely outcome of a repeatable process.”
Share on social: