6 tips for a successful deployment with an ML/AI vendor
Last updated on October 25th, 2022
Do you want to increase your production efficiency and product quality? Engage the services of a vendor that uses machine learning and artificial intelligence (ML/AI) on your manufacturing data. An ML/AI vendor can deliver focused, real-time visibility into your production and help you develop a data-driven action plan that will ensure improved quality and increased throughput.
Here are six tips to ensure a successful partnership with your chosen ML/AI vendor and their data scientists:
1. Set a clear and realistic goal
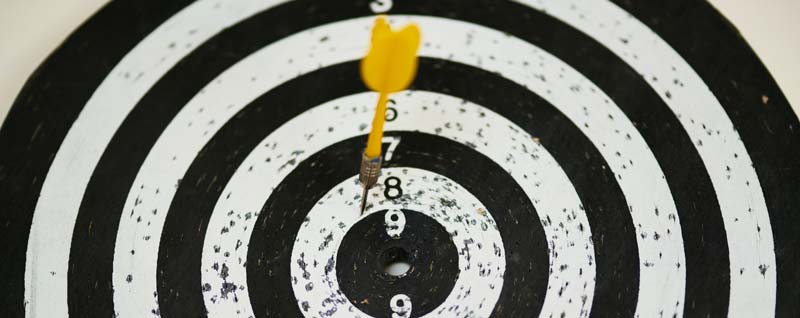
The first step to ensuring a successful partnership with your vendor is to have a clear goal in mind of what you want to accomplish. Then, make sure you provide accurate and relevant data to get the outcomes you expect. Below you’ll find two examples, illustrating the importance of setting a specific goal and providing the pertinent data to your vendor:
An example of the right way: A battery producer wanted to shorten their battery condition cycle. They needed a false positive to be a certain amount, and they already had a good understanding of their process. They provided specific criteria, which made it easy for the vendor’s data scientists to propose a viable production model for them.
An example of the wrong way: An automaker supplied their vendor’s data scientists with fleet-level data for all their vehicle models and wanted to know what predictive power their data had. They didn’t stipulate any specific feature or component, making it problematic for the data scientists to ascertain what they wanted out of the engagement.
2. Understand how to gather, manage, and label your data
It is important that you have a basic understanding of the landscape of your data. Knowing how to collect, handle, and label data properly, as well as how to set up a data model, are critical to the success of any engagement with your vendor.
For example: Mislabeling your data, especially when your project’s failure rate is very low, like in a warranty case, can lead to mistrust in the data output. If your data labels are wrong or the fidelity of your data is questionable, there could be a lack of confidence in the results.
3. Let the vendors talk to your data source
Sometimes it’s in your best interest to let your vendor’s data scientists communicate directly with your data source. The data scientists can get the information they need quickly and efficiently, verify the data to prevent delays, and avoid rework.
For example: Problems arise when data supplied by a customer is labeled differently to the way their vendor labels it, which causes a lot of back and forth before the data can be verified. This leads to delays in the exercise.
4. Make sure that your data is relevant
Once you have identified a problem that needs to be resolved, your vendor can develop a remedial action plan, using data insights from the information you supplied. It is critical that the data you submit is relevant to the problem that you’re looking to solve.
For example: An OEM was trying to solve a warranty problem pertaining to a specific component on a particular engine oil pump. Instead of supplying information relevant to that problem, they delivered assembly-level data expecting a targeted solution.
5. Provide a data sample prior to the engagement
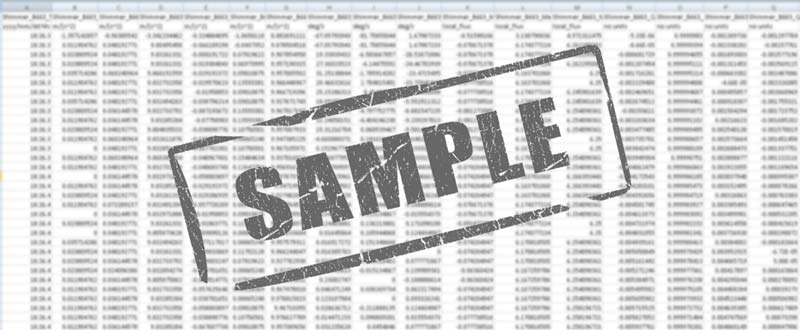
Get upfront feedback on the feasibility of your project by supplying your vendor with a sample of your data before the exercise. They can use the information to get a better understanding of your project and to create a proper statement of work (SOW) with accurate timelines.
For example: A major automobile manufacturer wanted to improve predictions for their end of line testing stand, but they didn’t supply any data samples. The vendor spent months working with the manufacturer’s data scientists to collect and link all the relevant data to build an improved end of line testing prediction model.
6. Have some domain expertise
Ensuring you have some basic domain knowledge will help you manage all stakeholders as your vendor goes through an exercise with you. A lot of your data science activities will go to your R&D team, who will then consult with your engineering and production teams, which can cause delays. Understanding the process of deployment will enable you to keep all parties informed and collaborating smoothly.
For example: A large auto manufacturer will likely have three or more departments involved in an exercise, and they won’t necessarily have the same goals. The R&D team is all about the future, the engineering team is all about the short-term, and the production team is all about now. You need some domain expertise to keep all parties on-track with the bigger picture and working together on data science exercises.
Ready to get started?
Having a productive partnership with your ML/AI vendor is key to managing insights into your manufacturing data and developing successful solutions. Acerta is at the forefront of improving production throughput and profitability with ML/AI. Leverage our domain expertise in precision engineering, data science, and ML/AI. Get in touch.
Share on social: