Can machine learning solve the last 5% in manufacturing quality?
Last updated on March 27th, 2024
Even though I’ve been working in the auto industry for more than decade, I’m not a car guy. But you don’t have to be a car guy to see the automotive landscape is changing. We could very well see more change over the next 10 years than we have in the previous 50, especially in automotive manufacturing.
What's So Special About Automotive Manufacturing?
One of the very first things I learned out of school was that manufacturing is a kind of know-how you learn from doing, not something you learn in a classroom. As important as university courses on manufacturing are, most line engineers will tell you their knowledge comes from being on the plant floor.
I’ve probably learned more from working with shop floor technicians than anyone, and I quickly came to trust their experience over what I’d memorized from books. They’re the ones who taught me what really sets the auto industry apart from other manufacturing sectors: a unique combination of aggressive productivity margins and demanding product requirements.
I’ve worked on assembly lines that crank out a part with over 30 components every seven seconds, and while some industries might be satisfied if those parts work 85-90% of the time, that’s nowhere near enough for automotive. Plus, the approach to quality is different at high volumes because it’s just not possible to check every feature on every single part or assembly; you don’t have the time for that luxury.
As my old engineering manager used to say, “It’s not easy to fix a plane while it’s in the air.”
Between the high demands on quality and the costs of stopping the line, you’d better be 100% certain before you take the line down to adjust something, because you’re going to hear about it from the production team. That’s why having more manufacturing data and better tools to analyze can have such a big impact on the auto industry.
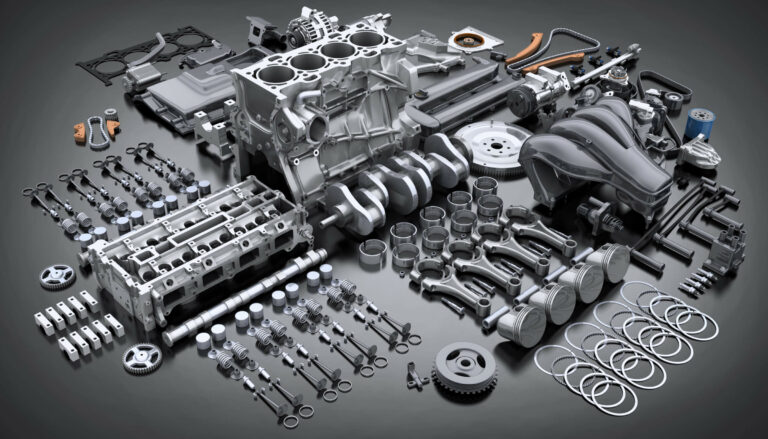
Manufacturing Data: Better to Have It & Not Need It...
I was collecting and aggregating data from assembly lines when I started out, but nowhere near the level of today. The modern assembly lines I’ve worked on have 2D matrices with birth histories for almost every sub-component, tabulating all the parameters for the operations they’ve passed through.
All of that gets written to an SQL database so that part and assembly data can be traced all through production right up to and including the End of Line test. Depending on how well it’s been integrated, that means traceability data all the way through production, which ends up being a lot of data. Of course, with diminishing IT costs, you might as well collect and store it if you’re already generating it. But what do you do with it?
In principle, it’s great to have all this manufacturing data, but a lot of manufacturers aren’t actually doing much with it. In many cases, they’re just using it to solve problems the same way they would have in the past: If you’re having a flare up today on a clearance check station, you can evaluate that trend in comparison to others. But that’s still taking the same reactive approach to solving the same problems.
If you want to do more with your manufacturing data, you need a new set of tools.
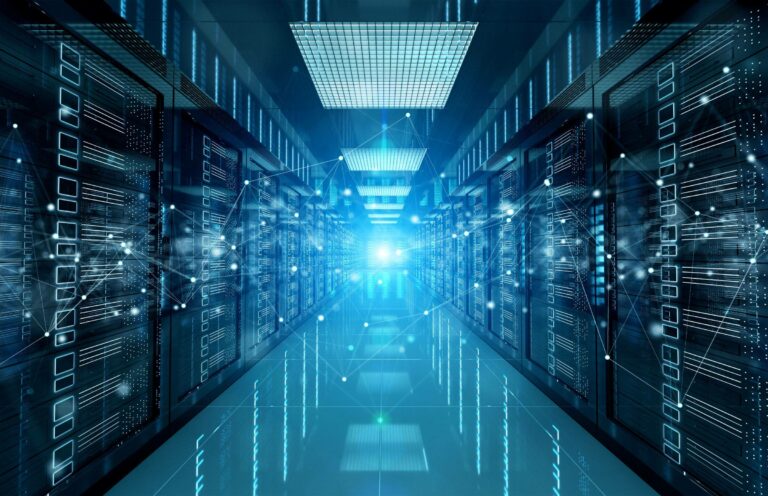
Machine Learning in The Auto Industry
The best way to leverage those huge volumes of manufacturing data is with machine learning. If we want to get that last 5% in quality, we can’t just look at production and testing data historically or reactively; we need to be proactive and use that data to build predictive models.
Once you’ve aggregated all your manufacturing data, you can start applying data science and machine learning to it. And when I say all of your data, I truly mean all of it. There are insights to be gained not just from average depth measurements but from collecting each individual LVDT measurement too.
Measuring torque or pressure response after solenoid actuation?
Record the time-series traces, not just the summary stats.
The human mind has always been very good at qualitative assessment—it’s second nature to look at two plots and comment on why they are different—but we have never been good at consuming large amounts of data. All that changes with machine learning, since we can now easily consume large amounts of data and make qualitative assessments by applying machine learning models.
Traditional root causing methods start having problems where there are many degrees of freedom and a daunting amount of feature interaction. Issues like NVH or EOL test performance problems are likely a function of several components and multiple features upstream. As a result, they cannot easily be discovered through traditional root cause analysis, but it’s this kind of application where machine learning really shines.
With machine learning in mind, automakers can set up assembly lines knowing what manufacturing data to collect and even integrate machine learning in the development phase. This could fundamentally change how we build quality and product control into new assembly lines, but first we need to get past the skepticism that data science and machine learning tend to evoke.
A lot of that skepticism comes from the way machine learning works. In looking at very complex relationships, machine learning models are hard to explain and understand. At Acerta, we work very hard on techniques like SHAP analysis so we can explain our models and approach to our customers and partners.
New Tools in Automotive Engineering
Engineers should see data science and machine learning as new tools in their toolkit. You might be making parts at 95%, but if you want to get that last 5%, you’re going to need something you don’t already have, and that’s machine learning. Obviously, we’re talking about an ambitious target, but I’ve never had a General Manager tell me that we’ll get to relax if we get to 85% OEE or 95% FTT.
It’s the companies and engineers that are willing to keep pushing after they hit these targets that are best positioned to embrace machine learning.
These tools can ingest and make sense of the reams of manufacturing data coming off today’s production lines. A machine learning model of the production process can help improve throughput and quality by enabling line engineers to understand the nuances of their lines and thereby identify and fix quality problems faster.
But that’s just the beginning.
Way back before my time, automakers assembled components using a selective fit methodology: workers would measure the cylinder bores on an engine block, for example, and then select the piston head that gave them the right running fit. That approach has fallen by the wayside for most applications because it’s not possible to dedicate that much time to each part.
Fortunately, with all the manufacturing data being collected, we can use machine learning to bring it back to high-volume production environments. Today, we can make better component selection decisions during axle assembly operations. Soon, an axle assembly with certain carrier and cover dimensions could be matched with an incoming pinion and ring gear set with complimentary properties to ensure a pass at the EOL test.
That’s what brought me to Acerta: while others are just starting to dabble in machine learning to see what data science can do for them, we’re helping automakers use those tools in real life production and on-road applications today. Compared to my colleagues, I still can’t say I’m really into cars, but I am into making them, and machine learning is the key to making them better.
Share on social: